Sixfold Content
P&C & Speciality
Meet Narrative: Your Shortcut to Risk Documentation
Sixfold’s latest launch introduces Narrative, a feature that helps underwriters document risk faster and more consistently. First rolled out with Zurich’s North America Middle Market team, Narrative is already helping standardize how risk is communicated across the organization.
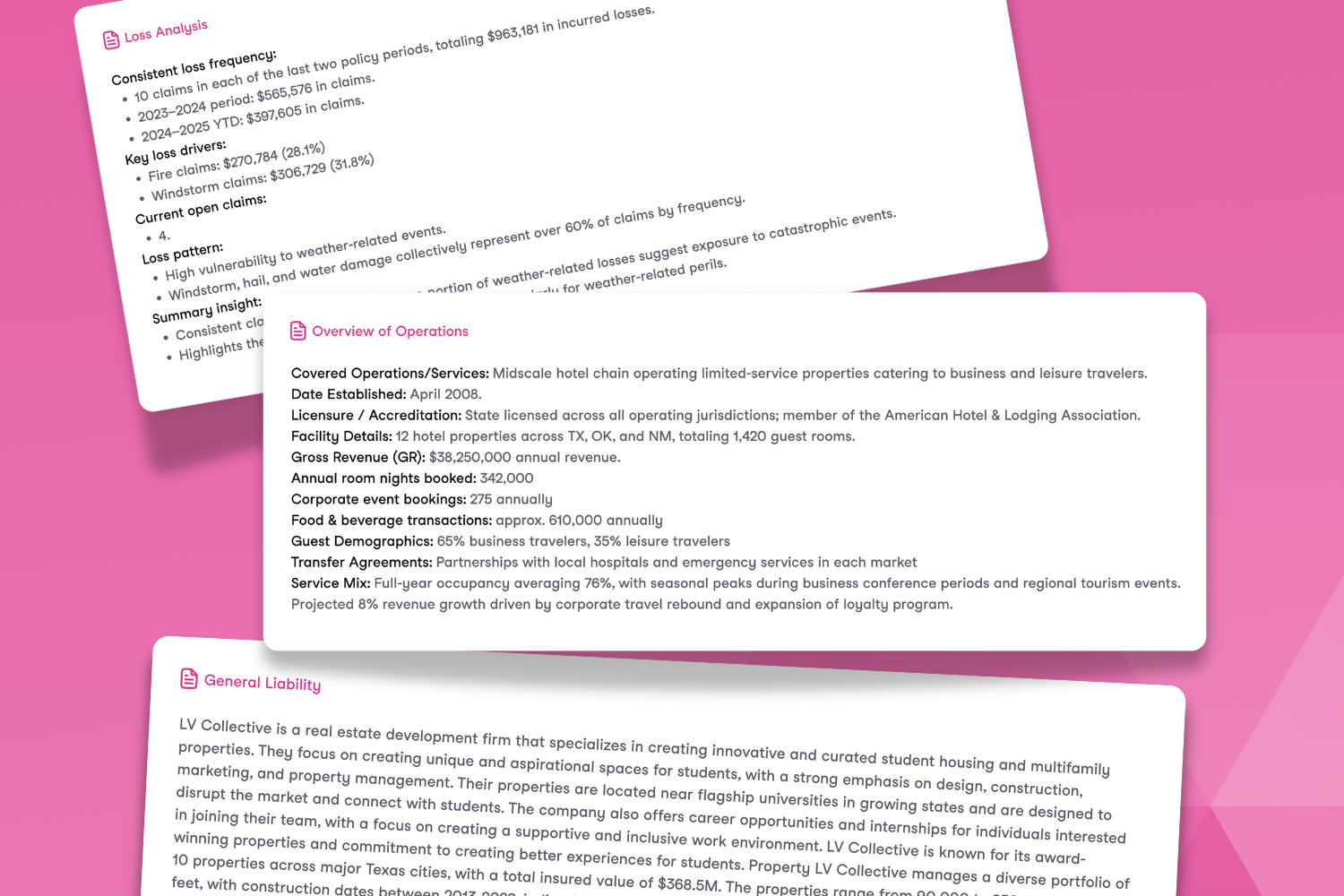
Explore all Resources
Stay informed, gain insights, and elevate your understanding of AI's role in the insurance industry with our comprehensive collection of articles, guides, and more.
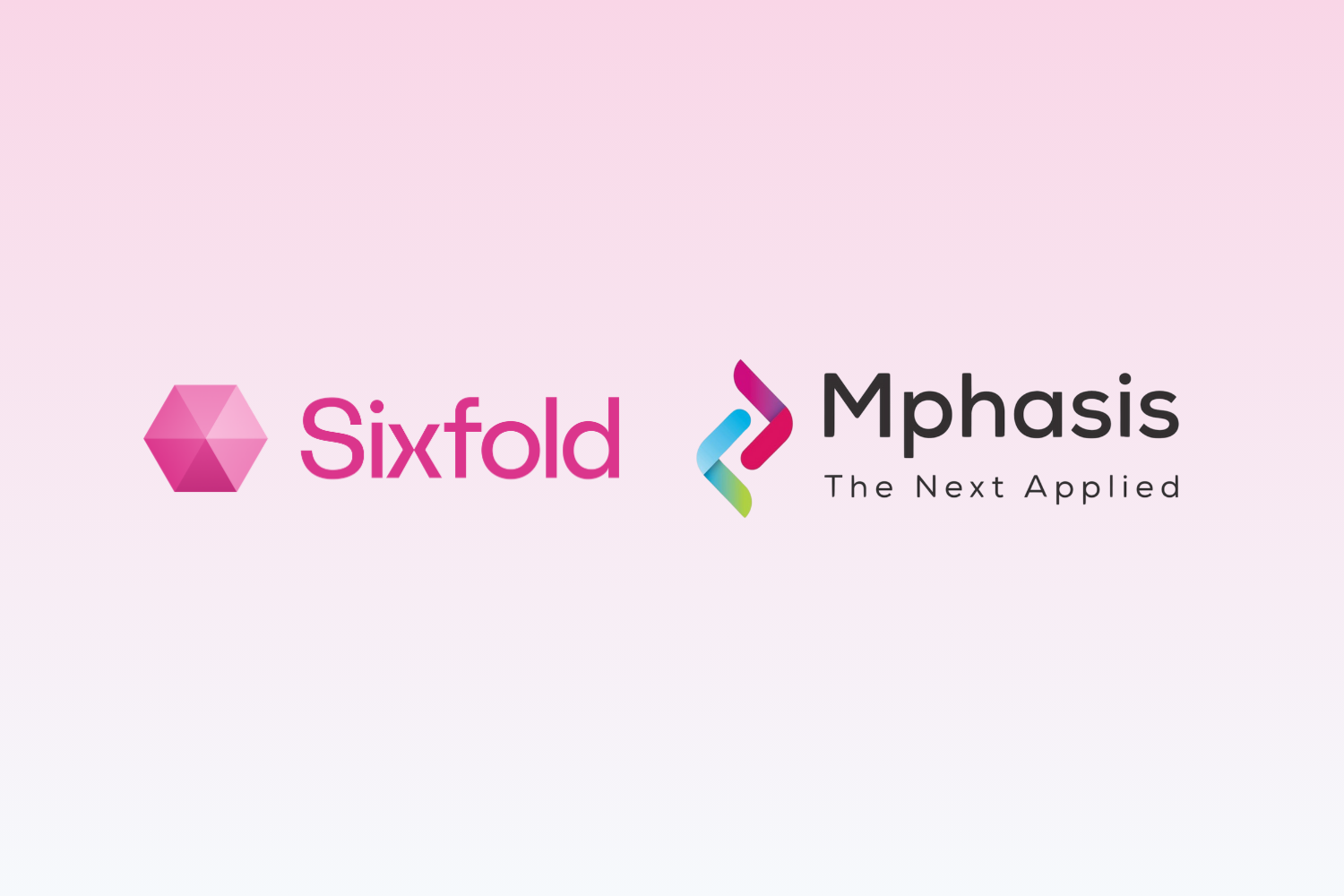
Sixfold Partners with Mphasis to Simplify Integration
Sixfold and Mphasis are teaming up to make AI in insurance underwriting faster to deploy and easier to use, helping insurers grow without delays.
Implementing new AI technology in your underwriting workflow can be a challenge. Between IT team bandwidth and integration complexities, getting a new workflow up and running smoothly takes time.
At Sixfold, we’re all about making underwriters’ lives easier and speeding up the quote process. So, of course, we want to make implementing our platform just as smooth and just as fast. One way to do that? Partner with experts who know operations & technology inside and out. That’s where Mphasis comes in.
Meet Mphasis
If you haven’t heard of them, think of Mphasis as a company that helps insurance carriers streamline operations, integrate technology, and make their day-to-day workflows simpler. Basically, they’re the experts at making complex systems work together, which makes this partnership a win-win for everyone involved.
Mphasis will integrate Sixfold’s platform to help carriers deliver faster, more confident quotes by giving underwriters contextual risk insights tailored to each insurer’s unique appetite.
“Mphasis is excited to partner with Sixfold to accelerate AI adoption in the insurance industry. By leveraging Sixfold’s AI expertise, Mphasis enhances its insurance technology capabilities to deliver advanced, data-driven automation solutions for global insurers, driving efficiency, accuracy, and innovation across the insurance value chain.”
- Nitin Rakesh, Chief Executive Officer and Managing Director, Mphasis
When it comes to new tech, speed to value matters and no one wants a slow start. With Mphasis, we’re making sure insurers can start seeing value from their Sixfold integration faster.
This partnership is about improving insurance underwriting and speeding up implementation, enabling insurers to scale quicker and stay ahead of the challenges in today’s market.
Read more about the partnership on Yahoo Finance, Life Insurance International and Fintech Finance.
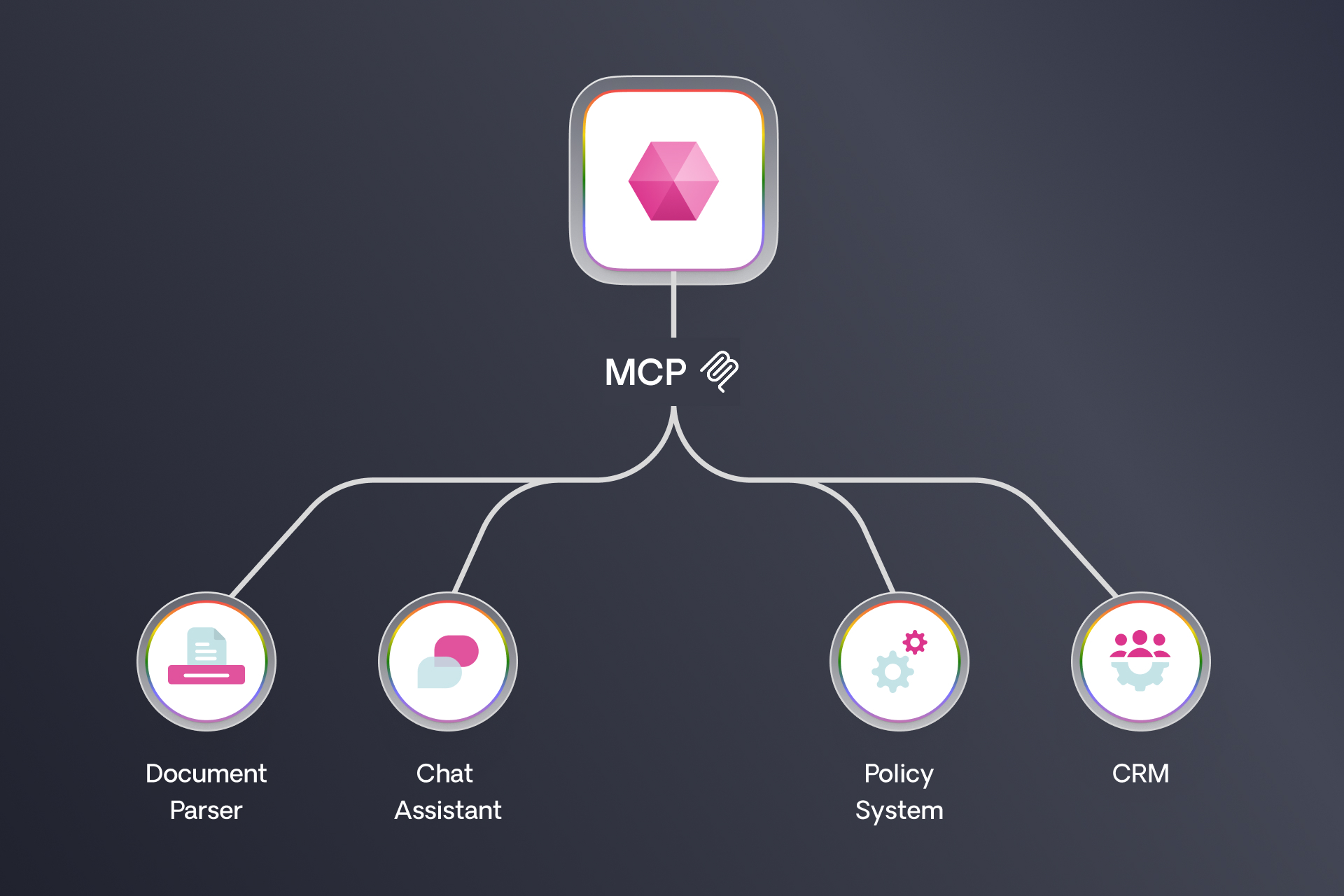
What is MCP - and Why It Matters for Underwriting
At Sixfold, we always integrate the latest AI advancements, but only when they truly help make underwriting faster, easier, and more accurate. One of the most promising technologies we’re exploring right now is Model Context Protocol, or MCP.
At Sixfold, we always integrate the latest AI advancements, but only when they truly help make underwriting faster, easier, and more accurate. One of the most promising technologies we’re exploring right now is Model Context Protocol, or MCP. Curious why? Read on.
What Is MCP?
MCP is a way for different AI models, and the agents (read about agents here) that use them, to talk to each other.
Think of it like this: instead of manually connecting different systems when you want to share data, MCP lets one AI model pull context from another in real time, seamlessly and instantly.
Basically, instead of teaching every AI everything, you teach each one what it’s best at, and they learn to ask each other for help.
Who’s Behind It?
Since its introduction, MCP has gained traction among major AI providers:
Anthropic: The creator of MCP in November 2024, Anthropic has integrated the protocol into its Claude family of language models, enabling them to interact seamlessly with external systems.
OpenAI: In March 2025, OpenAI announced support for MCP across its Agents SDK and ChatGPT desktop applications, facilitating broader adoption of the protocol.
Google DeepMind: Shortly after OpenAI's announcement, Google DeepMind confirmed MCP support in its upcoming Gemini models and related infrastructure, highlighting the protocol's growing industry acceptance.
...and many more! It’s not just the AI giants. Tools like Linear, Zapier and Atlassian are jumping on board. Signaling that MCP is becoming foundational infrastructure, not just something for the leading LLMs, but for the everyday tools teams use to get work done.
MCP + Underwriting AI?
Why is MCP relevant for AI underwriting technologies? Today, many insurers have their own internal AI tools. MCP basically turns all these isolated AI models into a connected ecosystem.

Here’s an example:
1. Let’s say a carrier has its own internal ChatGPT-like app as well as Sixfold's AI risk assessment solution.
2. With MCP, an underwriter using an internal chat tool, for example, can simply ask a question “What’s the risk appetite score for this customer named Gamehendge Widgets?”
3. Their AI doesn’t need to know everything itself, it just knows who to ask, in this case - Sixfold. It reaches out to Sixfold’s models, gets the answer, and serves it up directly to the underwriter.
No complex integration projects. No heavy lift for IT. MCP would act as a seamless bridge between Sixfold’s risk assessment expertise and the additional AI tools underwriters are using.
Why Sixfold Cares (a Lot)
Right now, almost everyone is talking about "agentic" behavior, how AI agents plan and reason independently. But the thing is that MCP is the quieter, more practical sibling: it’s about getting the right data in the right place, way faster than before.
The impact MCP can bring:
- It can meet underwriters where they are today - inside the tools they already use every day
- Enables flexible adoption where carriers can pull in just the capabilities they want, without a massive rollout
- It’s a leap forward in making underwriting AI more accessible and useful
At Sixfold, we’ve made MCP connections between our models and other systems, We’ve, and also exposed internal tools where AI chat assistants can query Sixfold’s underwriting insights.
What to Watch Out For
Like any new tech, MCP isn’t perfect. There are a few important risks to keep in mind:
Security:
- MCP is pretty quiet on the security mechanisms with which connected systems lock down their data. Existing enterprise-grade methods that companies like Sixfold use to protect sensitive customer data will need to be considered and implemented.
- Malicious tools could hide bad instructions if care is not taken on how different models talk to each other.
Accuracy:
- Incorrect or messy data leads to bad decisions. AI pulling data quickly doesn’t mean it’s always right, double-checking and validation are key.
What’s Next with MCP?
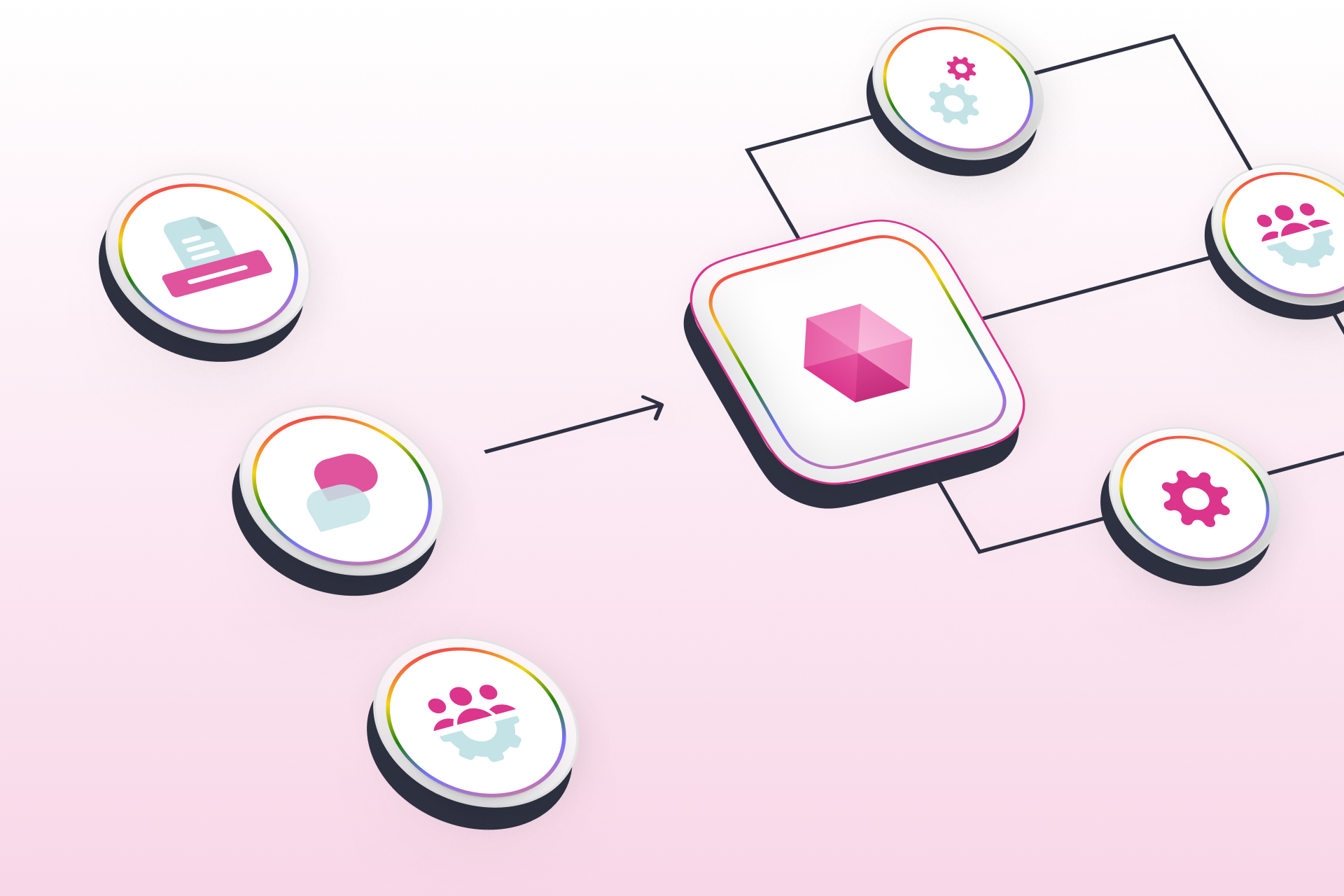
MCP done right (e.g. secure, validated and tested over and over) could be a kind of quiet but transformative technology that will make AI in insurance not just smarter, but more practical.
From many steps to one: MCP collapses complex processes into simple interactions.
From heavy integrations to light connections: Carriers can plug into any AI expertise easily.
From standalone tools to connected ecosystems: Underwriters get the best of all worlds, without even noticing the heavy lifting happening in the background.
Emerging protocol: Agent-to-Agent (A2A). A2A was launched by Google in April 2025 and has already gained support from Microsoft. The protocol addresses the need for AI agents, often developed on disparate platforms, to communicate and collaborate. Even with certain similarities, MCP and A2A work well together instead of competing. Google says that A2A is meant to go alongside Anthropic’s MCP.
As more organizations roll out MCP and A2A, AI assistants and agents are getting way better, more up-to-date, more capable, and way more useful in the flow of work.
The future of AI in underwriting isn’t just about smarter models, it’s about models that collaborate and talk to each other.
.png)
Sixfold’s UK Expansion: Q&A with New Regional Director
We sat down with our Regional Director for the UK to talk about her journey from underwriting to insurtech, what she’s seeing in the European insurance market, how AI is being adopted across the industry, and what makes this moment such an interesting one for insurers.
We were curious to get to know our new Regional Director for the UK, Rosina, so we sat down with her to hear how she got started in insurance, what she loves about the industry, her take on AI adoption in the UK, and more.
The takeaway? A conversation full of great insights.
Tell us about your career journey. How did you first get into the insurance and insurtech world?
I joined the Allianz internship programme in the UK during the 2011 England riots, and I was struck by the speed and coordination of the industry’s response. Until then, I hadn’t fully understood what a market event was. I was inspired by how ‘applied’ the actions happening in offices across the country were to real people and their unfolding losses.
What I had expected to be a theoretical application of my maths degree turned out to be something far more engaging, and I was gripped. I rushed back to join Allianz as soon as I graduated, spending seven years there in various underwriting, pricing, and data roles.
What I had expected to be a theoretical application of my maths degree turned out to be something far more engaging, and I was gripped. I rushed back to join Allianz as soon as I graduated, spending seven years there in various underwriting, pricing, and data roles.
What really stood out to me was the collaboration with data providers, academia, and at the time, a new breed of company: insurtechs. That inspired me to join the insurtech community myself, drawn by the opportunity to work with a broader range of insurers and the global reinsurance market.
What do you enjoy most about working in insurance? What is it about the industry that keeps you interested?
What I enjoy most is the sheer breadth of expertise across the industry. You have catastrophe modelers working alongside underwriters who know the ins and outs of something as specific as sprinkler systems.
I love seeing such a wide range of knowledge and perspectives come together to solve complex problems.
Tell us about your experience as an underwriter. Were there any pain points in the process that you think Sixfold can help solve?
I spent most of my underwriting career in the London property market, a fast-paced environment known for some of the world’s most complex risks. At the time, pricing algorithms could only take you so far. It was crucial to learn from the experienced underwriters around me. They approached risk differently. Somehow, they could spot features I couldn’t. Their insights often felt like magic, and I was fascinated by the way they saw things others missed.
Looking back, I realise that what I was trying to do is exactly what Sixfold does. Sixfold gives every underwriter access to the kind of insight you would expect from your most experienced peer, and it does that consistently for every case.
As I built up my own technical knowledge and kept the underwriting guide close, I also found myself asking a new question. How would those underwriters approach the risks I was looking at? What might they see that I hadn’t?
Looking back, I realise that what I was trying to do is exactly what Sixfold does. Sixfold gives every underwriter access to the kind of insight you would expect from your most experienced peer, and it does that consistently for every case.
You’re Sixfold’s first European hire, congrats! Based on your experience, how has AI already impacted the European insurance market?
AI has moved beyond the hype in the European market, with some successful implementations, especially in distribution and claims. There are admirable examples of AI improving customer experience and reducing the time to resolution. I believe these implementations have done a good job at building confidence and appetite for AI solutions across the market.
AI is now offering new value creation and will cause a rethink of some of our most fundamental beliefs about how insurance is designed. I think it is a really exciting time to be an insurer.
However, what is coming goes beyond enhanced solutions. AI is now offering new value creation and will cause a rethink of some of our most fundamental beliefs about how insurance is designed. I think it is a really exciting time to be an insurer.
How do you think European and North American insurers differ when it comes to tech adoption in insurance?
I think the distribution models contribute to some differences in tech adoption, and quite rightly so. In broker-driven and syndicated markets, there is a need to demonstrate benefit and build trust beyond the point of direct application.
This differs by business line, but in general, players who are able to run more effective A/B tests with their markets tend to move through the adoption process more quickly. Regulation also plays a part, with the EU and the US taking notably different approaches to early AI governance.
I think continued collaboration between European and North American players is vital to keeping that pace aligned with the expectations and emerging needs of the end customers we are all here to serve.
That said, in both regions, there are change makers willing to take first-mover risks, driven by a belief that the advantage will outweigh the early ambiguity. These change makers are setting the pace. I think continued collaboration between European and North American players is vital to keeping that pace aligned with the expectations and emerging needs of the end customers we are all here to serve.
How do you stay on top of the latest industry trends?
I am connected with various insurance networks, including the UK and US insurtech communities. I enjoy the insights that come from the conference circuit. But perhaps the most fruitful source of industry trends comes from the conversations I have with our insurance and reinsurance customers.
What excites you most about joining Sixfold?
I believe Sixfold, in conjunction with its industry partners, will help deliver the step change needed for insurance to meet the evolving needs of our customers and society more broadly. The vision here is not to change things for technology’s sake, but to use technology as a way to improve outcomes and experiences for both buyers and sellers of insurance, especially as they navigate shifting risks and changing consumer behaviour.
I have seen plenty of ambitious missions, but what excites me about Sixfold is its ability to keep both feet in the future of technology while staying grounded in the present. And I am thrilled to be joining a team made up of such high-calibre individuals.
I have seen plenty of ambitious missions, but what excites me about Sixfold is its ability to keep both feet in the future of technology while staying grounded in the present. And I am thrilled to be joining a team made up of such high-calibre individuals.
In your view, how does Sixfold stand out in this space?
What stands out to me is how dedicated to insurance everyone is. Walking into the Sixfold office feels like walking into an insurance HQ.
It doesn’t take long to see that Sixfold is working with some of the most exciting and advanced technologies out there.
What really stands out to me, though, is the focus on solving real industry challenges. In a way, it reminds me of my early experiences as an intern. This work goes far beyond the theoretical.

Just Launched: Instantly Spot Gaps in Medical Reporting
Sixfold’s AI for life and disability insurance is now able to automatically flag mismatches between what applicants report and what’s found in their medical records, giving underwriters a faster, more standardized way to catch inconsistencies before they become costly.
In life and disability underwriting, one of the most time-consuming and error-prone steps is verifying an applicant’s self-reported information.
Why? Because applications are long and detailed, and even when applicants are trying to be honest, omissions, intentional or not, are common. This is a growing concern across the industry, a recent Munich Re’s survey identified applicant misrepresentation as the most rapidly increasing form of fraud.
Sixfold’s Discrepancy Scan capability was built to address exactly this issue. Sixfold’s AI is now able to automatically flag mismatches between what applicants report and what’s found in their medical records, giving underwriters a faster, more standardized way to catch inconsistencies before they become costly.
When risk is hiding in the records

When someone applies for individual life or disability coverage, they complete a health questionnaire, like Part II, eMed, or a Med Supplement, disclosing conditions, medications, and history. From there, the underwriter kicks off verification: ordering APS reports, Rx histories, labs, and other third-party records.
As these often hundreds of pages of documents arrive, the underwriter is essentially playing detective — comparing what the applicant said to what the medical records reveal. Did the applicant disclose all relevant conditions? Are they taking medications they didn’t mention? Is there a difference in diagnoses or treatment history?
Underwriters dedicate significant time to identifying discrepancies because they are critical. A person's prescription history can reveal underlying health issues, sometimes even before a formal diagnosis is made. For example, a prescription for a weight-loss medication might indicate an associated morbidity.
Any inconsistency could signal fraud or simply an oversight. Either way, it matters.
See below for a quick product walkthrough with Noah Grosshandler, Product Manager at Sixfold.
The feature is currently focused on medications, but that’s just the beginning. We're planning to expand this capability to detect discrepancies across pre-existing conditions, procedures, family history, and lifestyle factors—always guided by what’s material to each insurer.
Minutes vs. hours of detective work
Sixfold’s new capability eliminates a critical bottleneck in underwriting. The traditional approach of manually reviewing hundreds of pages to spot inconsistencies is both time-intensive and susceptible to oversight.
The Discrepancy Scan changes that completely, surfacing critical discrepancies automatically instead. The result is a more efficient process where underwriters can confidently assess risk based on complete information, without the administrative burden of document comparison.
“Sixfold goes beyond summarizing medical histories, we spotlight the contradictions that can change a morbidity assessment. By drawing connections across medical records, we emphasize the most crucial facts for investigation.
This approach transforms hours of detective work into minutes, providing underwriters with confidence and efficiency in their decision-making processes.”
— Lana Jovanovic, Head of Product @ Sixfold
Get the full story upfront
Accuracy is everything in life and disability underwriting. With Sixfold’s automatic discrepancy detection, underwriters are able to get to a more accurate underwriting decision by:
- Catching omissions and inconsistencies at the beginning of the review cycle
- Reducing misclassification of risk due to overlooked or conflicting information
- Detecting potential fraud patterns before they result in costly claims
- Maintaining consistency and transparency when cases move between underwriters
How the feature works

The Discrepancy Scan automatically compares the self-reported application data against the supporting medical documents and flags any mismatches related to material facts.
Prescriptions are often a leading indicator of an underlying diagnosis, one that could directly impact insurability or rating decisions. But not every medication matters the same way, and what’s considered “material” varies from carrier to carrier.
By securely ingesting each carrier’s unique underwriting guidelines, Sixfold identifies which medications are truly relevant in each context, connecting the dots between prescriptions, diagnoses, and underwriting impact.
Here’s how the feature works in practice:
1. Medical Document Review
Sixfold’s AI reviews both the submitted application and any supporting documents uploaded (APS, MIB, Rx histories, etc.) for medical data relevant for risk assessment.
2. Discrepancy Detection
Sixfold then compares the findings in the medical documentation to what the applicant reported. If a medication appears in the documents but not in the application, it’s flagged as a discrepancy.
3. Discrepancy Alert
Within the underwriter's dashboard, discrepancies appear clearly labeled with clear icons. Clicking into a card brings up the relevant context e.g., “Blood thinner mentioned in the medical report, not disclosed by the applicant.”
4. Clear Next Steps
Underwriters can use this insight to request clarification from the applicant or additional documentation from providers.
5. Always-Current Monitoring
Because documents arrive asynchronously, the system continually updates as new files are uploaded. Discrepancies are flagged dynamically based on the most current information.
Learn More
Insurtech Insights takes a closer look at the Discrepancy Scan
Interested in a hands-on demo? Reach out for a Sixfold walkthrough

Sixfold’s Take on Agents
Not sure what an AI agent is? You’re not the only one. We chatted with Sixfold CTO Brian Moseley to explain what agentic AI actually is, why it’s suddenly everywhere, why it matters for underwriting, and how we’re using it at Sixfold.
Not sure what an AI agent is? You’re in good company. We sat down with Sixfold CTO Brian Moseley to unpack:
- What “agentic AI” really means
- Why it’s suddenly everywhere
- Why it matters for insurance underwriting
- How we’re putting this technology to work at Sixfold
The Definition Dilemma
What exactly are agents in the context of AI?
That’s harder to answer than it should be.
The industry hasn’t coalesced around a standard definition. According to swyx, a software engineer might say that an agent is “LLM calls in a for loop.” Pretty reductive. If you buy them a beer, they’ll probably start talking about tools, memory, and LLM-based control flow - fascinating but deeply technical concepts that don’t offer much pragmatic insight.
Basically, an agent can accomplish a complex task by choosing how to progress through a complex, evolving process without ongoing human input.
For my money, Addy Osmani offers the most useful working definition. He says that “AI agents [...] are autonomous systems designed to perceive their environment, make decisions, and take actions to achieve specific goals, all while maintaining context and adapting their approach based on results.” Basically, an agent can accomplish a complex task by choosing how to progress through a complex, evolving process without ongoing human input. Contrast this with the typical chatbot interaction, where a human submits a sequence of one-shot requests.
How do you define the difference between a workflow and an agent?
The key difference is that a workflow is consistent and predictable. Given the same set of inputs, it will proceed the same way every time. A workflow can be dynamic, with conditional or looping steps, but it is inherently deterministic.
An agent’s process is driven by probabilities. Even if given the same inputs twice in a row, an agent may choose a different plan of attack each time and will almost certainly generate different outputs on every run.
An agent’s process is driven by probabilities. Even if given the same inputs twice in a row, an agent may choose a different plan of attack each time and will almost certainly generate different outputs on every run. You can reason about what is likely to happen, but there are no guarantees. You have to be comfortable with a range of outputs and have some tolerance for error.
The flexibility is exactly what makes agents so powerful, and why they’re now at the center of so many AI conversations.
Why Everyone’s Talking About Them
Why do you think agents have become such a hot topic?
Businesses are always looking for ways to do more with their resources. First, we automate repetitive tasks that are consistent and predictable from one execution to another. But once those possibilities have been exhausted, the next leap is enabling software to handle more complex, reasoning-based work.
If this is successful, we can offload even more, not just the rote work that is often done on autopilot, but also the tasks that require real thinking.
If this is successful, we can offload even more, not just the rote work that is often done on autopilot, but also the tasks that require real thinking. That’s the promise.
But it gets even better. We can envision a world where, as the cost of intelligence is driven down, every worker becomes a manager of a team of agents, setting goals, reviewing work, testing many ideas simultaneously, and picking the best with high confidence. This goes beyond improving productivity — it’s evolution.
Where Agents Fit Into Underwriting
Where do you see agents making an impact in underwriting?
The underwriting process itself is relatively structured and rules-based. But within that structure, underwriters deal in nuance, like recognizing when a 10-story building without sprinklers is still acceptable due to fire-resistive construction and strong safety measures, or approving a contractor engaged in high-risk work because of rigorous safety protocols and certified staff. They have to understand a risk so completely that they can craft a very thoughtful quote and risk management solution for the customer.
The underwriting workflow itself might remain orchestrated traditionally, but each step, the "needle-in-the-haystack" moments, could be powered by intelligent agents making context-based decisions.
That’s where agents come in. The underwriting workflow itself might remain orchestrated traditionally, but each step, the "needle-in-the-haystack" moments, could be powered by intelligent agents making context-based decisions. And instead of one underwriter doing all the work, an entire network of specialized agents can be turned loose on the risk to each solve a specific, context-rich problem, then synthesize the findings.
Agents at Sixfold
How are we using agents across Sixfold today?
The most obvious starting point is our Q&A feature. It starts with pre-set underwriting questions, scanning applicant data to find the answers, and summarizing them for the underwriter. While the feature began life as a simple one-shot LLM interaction, it has evolved over time. Now, if the input is complex, the system breaks it down into simpler sub-questions, answers each separately, and then summarizes those outputs into a final response.
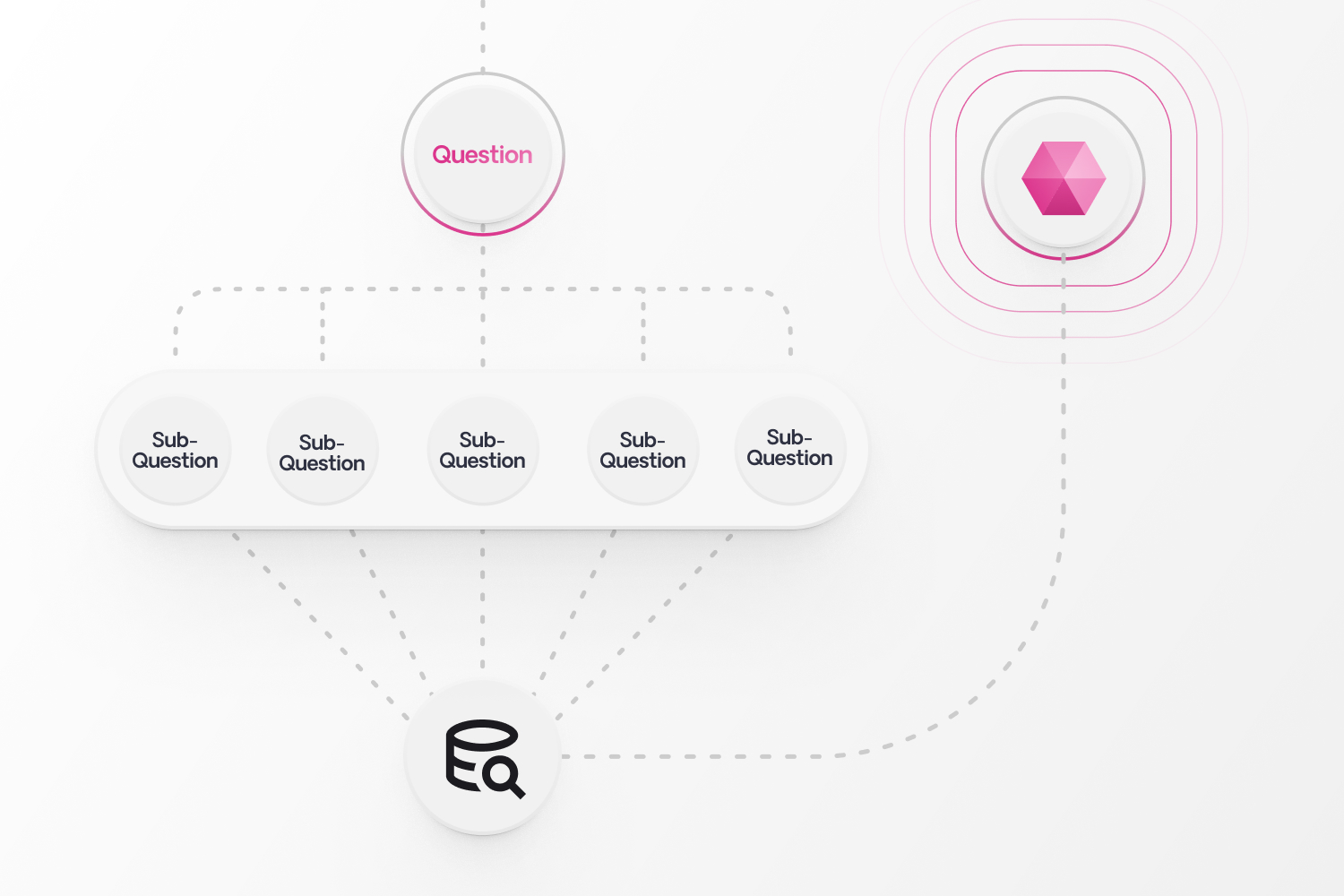
Next up is our Research Assistant, an agent that performs targeted web searches depending on the context of the business. For a given question, it decides how to search, what sources to consult, and how to rank results. We anticipate the research assistant will boost our ability to answer 30% more critical questions, giving underwriters a more complete understanding of risk before quoting.

We’re researching ever-more advanced techniques for document extraction. We’re experimenting with agentic approaches to classifying documents and routing to customized pipelines that can better extract information from complex structured documents like loss runs—use cases where traditional methods often fall short.
The Future of Agents
Are there limitations to agents today?
Technically, we’re still in the early days. As discussed earlier, we can’t even agree on a shared definition yet. And the methods by which agents interact with their environment (MCP) and each other (A2A) are evolving quickly.
But there are plenty of tools that help engineers build and deploy agents, singly or in swarms, and the landscape is rich and competitive. Amazing engineers like Simon Willison are doing yeoman’s work to help us understand the new models, tools, and techniques that pop up every day. It’s a great time to be a builder!
I think the bigger challenge is societal. What does the world look like when every person has a thousand agents at their fingertips? How will we experience the changes that advance us to that point? What will work - and leisure - look like?
Are there historical tech shifts you’d compare this to, like the internet or the iPhone?
Those were 10x changes, but what we’ve seen and will see in the five years after ChatGPT feels like 100x or even 1,000x. I don’t think anything else in modern history really compares.
There’s a lot of agentic hype right now, and some of it feels over the top, like the idea that AI will be writing most code in just a few months. You have to take some of this stuff with more than one grain of salt.
At the end of the day, it has to make sense for underwriters. We’re all about using the best tool for the job, regardless of what everybody happens to be talking about at the moment.
But overall, this feels different. Even if I’m naturally skeptical about the flavor of the day,I think there’s something real and lasting here, and we are leaning heavily into it at Sixfold. On top of what I mentioned earlier, we’re working through several new concepts that could make it into our roadmap.
That said, we’re not doing agents for the sake of it. At the end of the day, it has to make sense for underwriters. We’re all about using the best tool for the job, regardless of what everybody happens to be talking about at the moment.
References
latent.space/p/agent
docs.google.com/presentation/d/1SWoBIvTQu__uNEvSawmNcROiUx-n86O_fP0arZcTGb8/edit#slide=id.g2d9839ccb1c_0_0
addyo.substack.com/p/what-are-ai-agents-why-do-they-matter
huyenchip.com/2025/01/07/agents.html
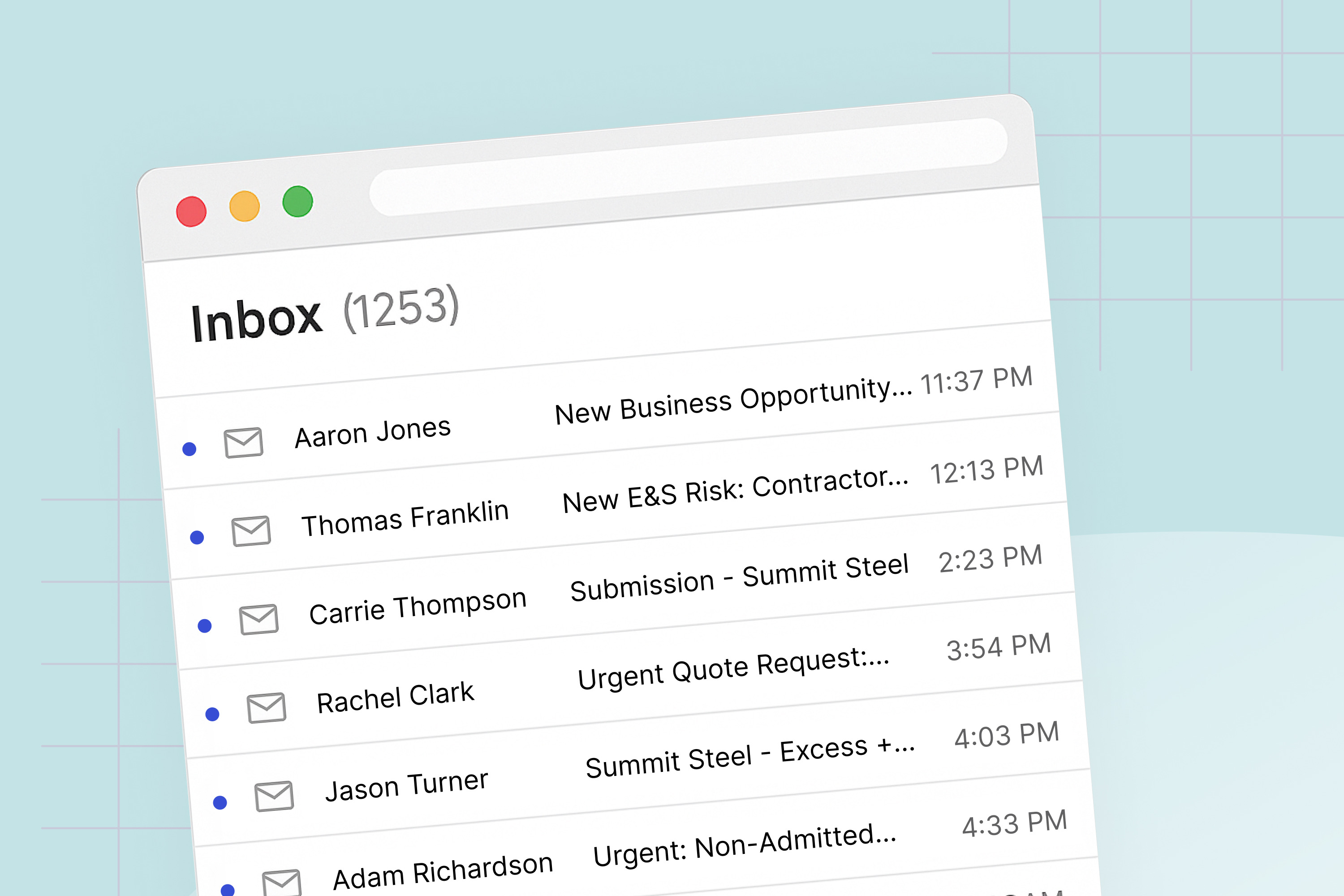
If You’re Not Fast, You’re Late in E&S
E&S insurance demand has surged over the past few years, and for underwriters, that means more cases to sort through every day. The solution? Quickly identifying the your relevant risks.
Excess & Surplus insurance demand has surged over the past few years, and for underwriters, that means more cases to sort through every day. We’ve heard from professionals across the segment about how high the case volume has gotten and how challenging it is to keep up.
So what’s driving this? We’re talking about one of the fastest-growing lines in insurance, with a 21% compound annual growth rate over the past five years, according to Insurance Journal. The growth comes from the segment’s ability to handle uncertainty like economic shifts or environmental changes, which have gotten more complex in recent years.
We’re talking about one of the fastest-growing lines in insurance, with a 21% compound annual growth rate over the past five years
One example is a cannabis business trying to get insurance. That kind of risk wouldn’t even be considered a few years ago. Since E&S takes on the risks standard carriers won’t, it’s absorbing more business, especially as admitted carriers get more cautious with the economic changes. At the same time, wholesale brokers—experts in hard-to-place risks—are sending more business than ever to E&S carriers.
The market's growth has also led to increased participation from both newly-capitalized and re-capitalized insurers, as well as managing general agents entering the distribution side. This influx has intensified competition, resulting in a decline in market share for 14 of the top 25 E&S players in 2021, according to Risk & Insurance.
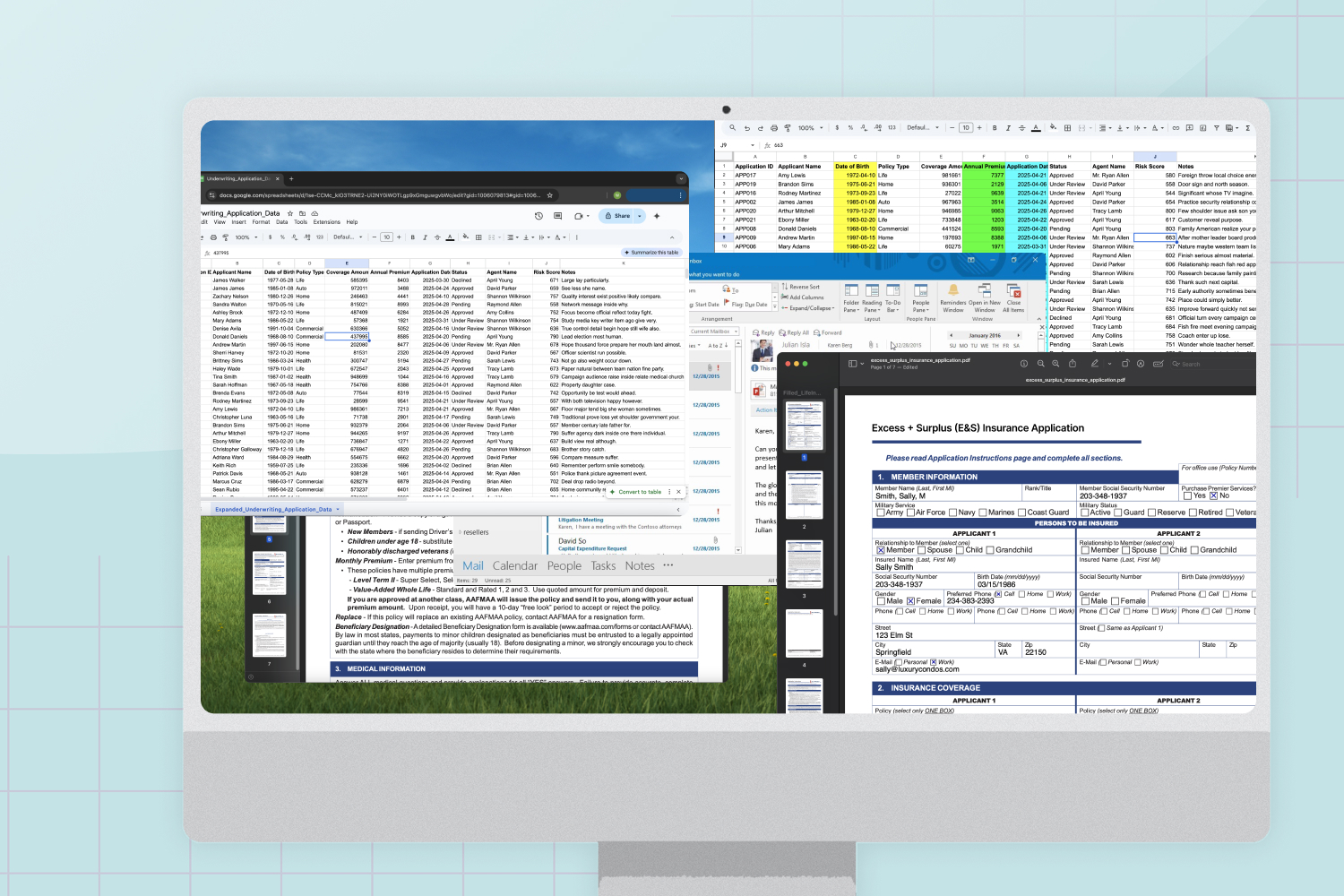
The Hidden Bottlenecks in Quoting
As the market surges, underwriters are buried in rising case volumes and more complex risks. With more players, competition, and pressure from wholesale brokers, getting quotes back quickly isn’t just nice to have–it’s critical for winning and retaining business. But what is currently causing delays in the quoting process?
As the market surges, underwriters are buried in rising case volumes and more complex risks
1. Many submissions, no easy way to filter them
E&S carriers receive all the non-admitted risks that standard insurers decline, often from a wide range of wholesale brokers. That volume adds up fast. The immediate impact? Underwriters have a hard time identifying the winnable opportunities and spend time working on cases that are out of their appetite.
2. Quoting process is mostly manual
A big part of the quoting process is manual. There’s a lot of data entry, systems that don’t help with prioritization, and information in all kinds of formats.
Because of that, E&S underwriters are still spending a lot of valuable time on manual tasks. In some cases, quoting can take up to 30 days. Think about incorrect routing between departments, incomplete information from brokers, and offshore teams handling SIC/NAICS code classification.
All of that slows everything down. And brokers? They’re expecting fast answers.
And brokers? They’re expecting fast answers.
3. Complex risks with tight deadlines
E&S risks are complex, and they take time to quote. But with the amount of submissions coming in, there’s just not enough time in the day to go through them all manually.
Underwriters need fast access to the key information that actually matters. That’s the only way to speed up quoting or quickly say no to risks that don’t fit.
The Result
All of this leads to premiums not being looked at, slower response times to brokers, and losing good deals to the competition. In a segment where demand is high and it’s nearly impossible to assess every risk, it’s key to spot high-quality submissions earlier in the process so carriers and underwriters aren’t stuck spending time on risks that won’t bind.
And when that happens, it’s not just GWP left on the table—it’s lost time and momentum.
Just think about the amount of premium carriers could capture by identifying the right opportunities from the start. Especially in a market that reached $130 billion in direct premiums in 2024 according to Insurance Insider US.
Imagine recieveing 50 submissions and already knowing which ones to prioritize, which ones fit your guidelines, what’s worth pricing creatively, and what’s a fast no
So, how can underwriters identify the right risks?
It all starts with quickly understanding whether a submission matches your appetite through an efficient triaging process. Imagine receiving 50 submissions and already knowing which ones to prioritize, which ones fit your guidelines, what’s worth pricing creatively, and what’s a fast no.
It’s not about writing any piece of business, it’s about writing the right ones for your business. With proper triaging, underwriters can move faster, get back to brokers quicker, and focus on what matters.
So, how do you actually get to the right risks faster? That’s where Sixfold comes in.
AI that Instantly Identifies Your Top Risks
Sixfold’s triage solution speeds up decision-making with instant, appetite-aligned scoring. Here’s how it works:
1. Showcasing the cases you want to quote
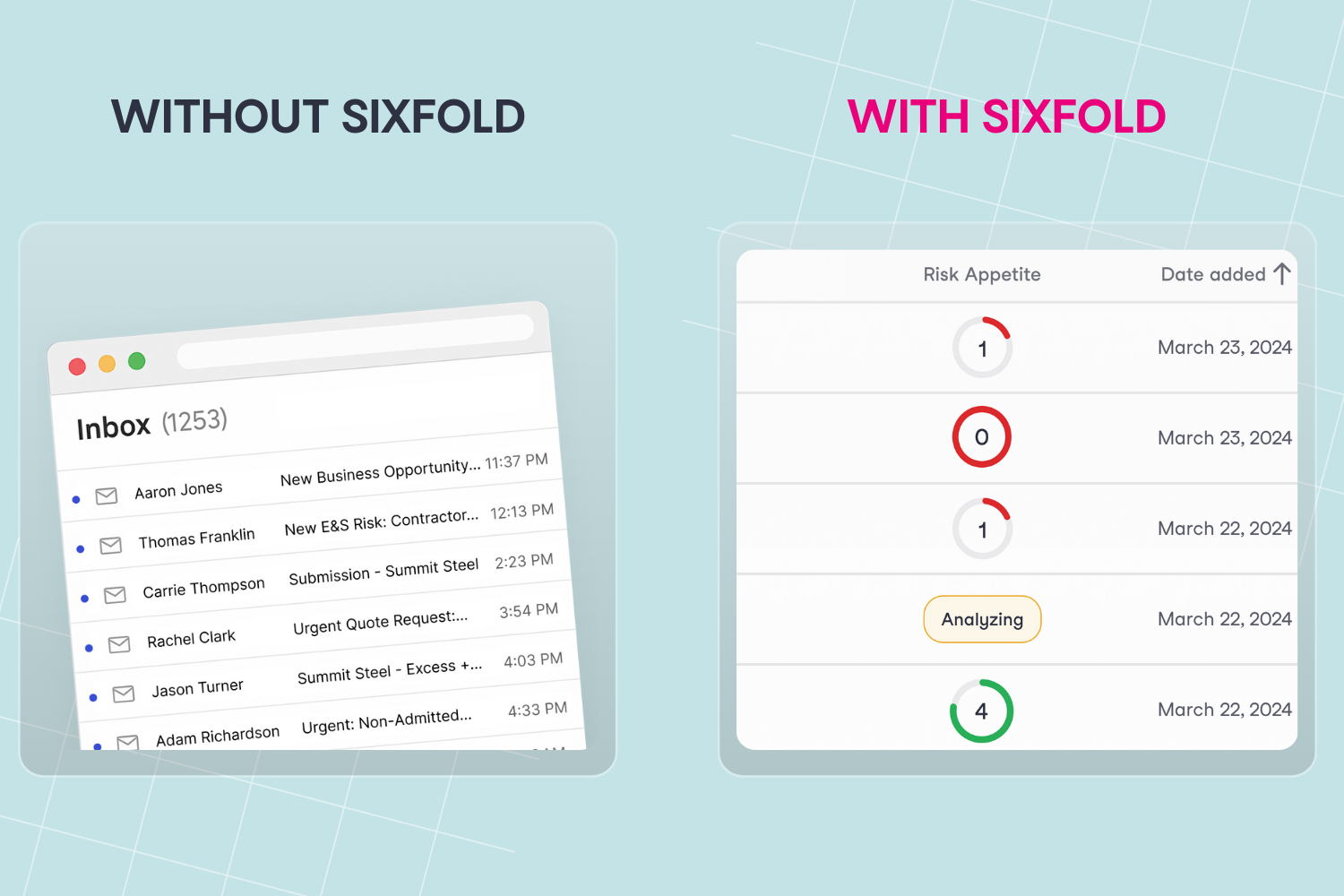
Sixfold ingests each insurer’s underwriting guidelines to learn the company’s unique risk appetite. Then, based on SOVs, applications, loss runs, and additional data, Sixfold’s AI runs the risk assessment, using both the documents uploaded and relevant company info it pulls from the web. It looks at the data points that matter for the insurer, such as for example construction year, occupancy, loss history, and more. From there, it generates a risk score for the submission from 0 to 5.
- 0 means it doesn’t fit your risk appetite at all
- 5 means it’s a highly qualified risk for you
Solving the front door issue by filtering risks immediately means underwriters can respond faster, whether it’s a quote or a decline
The impact? Underwriters know right away which incoming applications are worth their time. Wholesale brokers are strategic partners for E&S carriers. But when underwriters get too busy, they are sometimes left waiting for a reply. Solving the front door issue by filtering risks immediately means underwriters can respond faster, whether it’s a quote or a decline.
2. Classifying businesses with > 90% Accuracy

In E&S, sometimes a small difference in business activity can immediately make a risk fall out of the risk appetite. That’s why accurate NAICS and SIC code classification is key.
Sixfold automatically matches each submission to the correct business classification code, even for highly nuanced and complex industries, so no more time is wasted trying to figure out what type of business the company is. This supports better routing and faster underwriting decisions.
3. Presenting contextual risk factors

Sixfold surfaces the risk signals that matter most, whether they disqualify a submission, negatively impact it, or strengthen it. Everything is aligned with the insurer’s appetite and focused on the factors that drive the overall decision. Underwriters get precisely what they need to make confident calls.
Underwriters get precisely what they need to make confident calls.
See It in Action
The volume of submissions in E&S isn’t slowing down. But with the right triage process, underwriters can focus on decision-making, quote the right risks faster, and bring in more premiums.
The carriers winning today aren’t working harder; they’re triaging smarter.
Join our E&S product demo on May 21 with Alex Bontz, Customer Success Operations & Growth Lead. He’ll walk through how Sixfold quickly triages complex submissions and delivers the key risk insights underwriters need to take action.
Looking to catch up in person? Come find us at the E&S Reuters Conference on May 28. We will be there to connect with insurers looking for ways to improve their underwriting process with purpose-built AI.